Currently, when it comes to experimentation, the default is A/B/multivariate testing (testing different variants live on a subset of real users). As helpful as both methods can be (and they really are), A/B/multivariate testing both have issues. The big one being, the majority of companies don’t have the budget to wait weeks for a test to run its course only to achieve statistically insignificant results. We think this is where simulators can help as a way to prequalify product ideas with synthetic users.
What is synthetic data?
Synthetic data is computer-generated using artificial intelligence rather than being created from real-life events. It’s often modeled on an original real data set and made to mimic the same characteristics and structure of the original data.
Some of the benefits of synthetic data:
- Helps preserve the privacy of real-world data
- Can supplement insufficient data
- A cheaper alternative to collecting real-world data
What are synthetic users?
These are user profiles which have been AI-generated. There are different methods for creating these archetypes, for example, a pure Large Language Model (LLM)/AI chatbot approach trained on available data about people - demographics, lifestyle habits, behaviors, preferences etc. but it does raise questions about accuracy. An answer to accuracy issues is to take a hybrid approach, combining GenAI with underlying behavioral frameworks and deep learning models, trained on internal zero- and first-party data.
Blok’s approach is to tackle some of the issues with product experimentation by creating a simulation environment with synthetic users. We create synthetic user profiles modeled on your real-life user base, taking into account product activity, real customer feedback, and behavior research.
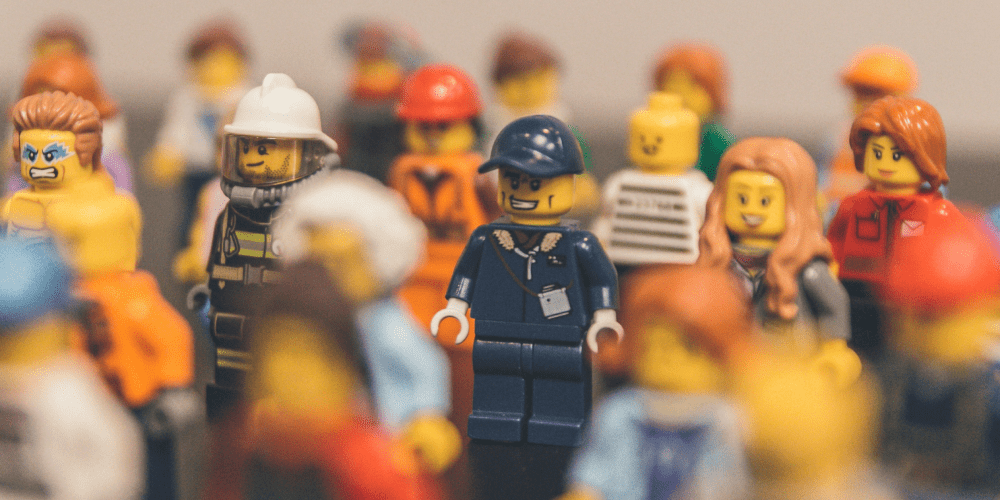
The benefits of simulators for product decisions
Save time and money
When simulating experiments, you don’t have to wait weeks for results. This is particularly useful for experiments which have a long Time-to-Insight period. A simulator can help give initial signals for discussing internally with the team - helping as a sense-check to get buy-in from engineers and leadership . It can especially work well as an assist to live experimentation where you can run preliminary experiments to help prioritize the most promising tests before deploying live.
Beyond A/B testing, which relies on first-party data around in-app behavior, simulating product changes with synthetic users also helps overcome barriers to zero-party data collection, such as surveying and customer feedback sessions. A simulator shouldn’t replace talking to your users directly, but it can reduce the burden, particularly for iterative changes or for simply soundboarding initial assumptions. Barriers to gathering research data can come down to cost, time and even issues around confidentiality. With synthetic data, you’re also saving on the regulation costs around data privacy and security which come with handling real-life data.
Another great benefit of synthetic data is that it can help supplement and scale existing real-world data to produce large datasets. This is great for data-hungry AI models and helps to democratize the ownership of the technology, giving smaller teams and startups the ability to compete with big enterprises.
Greater flexibility
Working with a simulator means you can play around with what-if scenarios by manipulating variables such as user preferences to include future segments you might be looking to target and even the wider parameters of the simulation environment such as market conditions.
For product development, synthetic users can also become a part of the development process for soundboarding changes at different stages. Whereas, the more costly alternative to avoid testing anything unpolished live on your user base, would be to recruit focus groups. You can also flip things and learn from the bad. In a simulation environment, you have the ability to purposefully run a bad experiment to discover insights you might not have otherwise uncovered - all without annoying your real-life users.
Reduce risk
In A/B or multivariate testing it’s very normal to churn through lots of alternative experiment variants, before landing on the right one and learning by failure is a standard part of the process. Putting aside the cost aspect of this approach, there is also the risk of a bad variant making it in front of your real-life users. This is where exploring live with real users, can end up negatively impacting the user experience and ultimately damage sales and KPI’s. This is where involving a simulator in the product development process can help mitigate these risks, by first testing changes on synthetic users and modeling out your assumptions.
Working with synthetic users instead of real-life users is particularly beneficial for high-risk environments where changes can have an outsized impact. For products which deal with sensitive topics, synthetic data offers greater privacy for example, generating artificial medical histories in healthcare. Basic anonymization isn’t enough to protect the privacy of your users since you can connect the dots and piece together someone’s identity (anyone who saw the allegations against Netflix for its show Baby Reindeer will know this). Using synthetic users, you’re able to offer a realistic alternative - mimicking the underlying structure and features of real data without linking back to real people. So product teams can carry out research without revealing the preferences of a single individual user.
Synthetic data has its benefits, but it does run the risk of being inconsistent with real-world data. Thankfully, nowadays, there are approaches to synthetic data generation that more accurately mirror the statistical properties of real-world data, closing the gap between simulated and real-world results. And the more real-world inputs you have the better - a great complement to surveying and feedback interviews whilst cutting down on the overall cost of research. Interestingly, in some circumstances, synthetic data can outperform real-life data. There are several reasons for this but it can be down to bias during the collection process or down to the processing of the data for example, human error when labeling data before being fed through an AI model.
If you're interested in how you can use a simulation environment for your product experiments, you can book a call with us here.
Header Image Credit: Maxim Hopman