The HIPPO Principle
When it comes to prioritization, many teams default to the HIPPO principle, otherwise known as the “highest paid persons opinion”. This is where decisions are made top-down in an organization. There’s the age-old battle of the visionary versus the incrementalist, or the Steve Jobs versus Mark Zuckerberg leadership style.
- Steve Jobs vs Mark Zuckerberg Leadership
Steve Jobs was known for his secret projects, big vision ideas and grand product reveals to beat competitors to market. Mark Zuckerberg, was known for his data-backed, agile approach with MVP’s and measurable decisions.
As with most things, balance is key. Accelerated by the recent wave of GenAI, there's a race to be at the cutting edge. Many are falling back on the ‘Steve Jobs style’ of big revolutionary ideas to help their companies stay competitive. There's a place for big visionary bets, bold swings and shiny tech showcases to get consumers excited but that’s only half the battle. We’re already seeing the pushback as companies integrating AI solutions are wanting to see ROI on these bets.
Not only this, but technical moats are no longer the deterrent they once were - as AI adoption naturally increases, competitive advantage diminishes. A bold vision is needed to help steer the company but when tech is no longer the differentiator and everything is being seen as disruptive, there is a growing need for a more tactical approach.
It also creates a greater need for distribution. The issue with distribution though, is the old ways are no longer effective with the rising costs of digital ads and the loss of web cookies. This is where we're seeing product-led growth (PLG) having its moment. This bottom-up approach is more aligned with the Zuckerberg style and puts product data in the driving seat for user acquisition and retention. The data-driven approach of product experimentation, democratizes decisions away from one all-knowing figure head, giving everyone within the organization the power to identify opportunities and contribute to the growth of the company. Not only does this help distribute the cognitive load but opens decision-making up to a greater diversity of perspectives and allows the space for a fellow team member to spot something that the visionary leader might not.
RICE and ICE Frameworks
Of course, when it comes to product experimentation teams can’t test every possible idea. Many use either the RICE or ICE framework. These are assessments product managers and teams can carry out to help quantify decisions around which features and product ideas to prioritize.
The RICE Framework
The RICE method, is calculated by the formula: (R x I x C) / E
(R) Reach - how many will see these changes (measured in number of users or customers)
(I) Impact - estimated impact on metrics (0.25 = minimal impact, 0.5= low impact, 1 = medium impact, 2= high impact, 3= massive impact)
(C) Confidence - level of confidence in your estimates for Reach, Impact and Effort (scored as a percentage e.g. 50% is considered low confidence, 80% medium, 100% high)
(E) Effort - cost in labor and time (scored in number of people x time in months e.g 3 people needed for 3 months = effort score of 9)
The ICE Framework
For the ICE method, each component is scored on a scale from 1 to 10. To calculate your ‘ICE’ score multiply I x C x E
(I) Impact - estimated impact on metrics
(C) Confidence - level of confidence in your estimates for Impact and Ease
(E) Ease - how easy to implement both in terms of effort and resources needed
As much as these frameworks help to simplify decisions, they're subjective and you can easily end up with different scores depending on who is carrying out the assessment. So, despite their formula-based framework, they can feel somewhat made up as they're still rooted in gut decision and opinion. Often you'll end up in lengthy discussions with everyone pushing for their own idea, peddling massaged evidence alongside it.
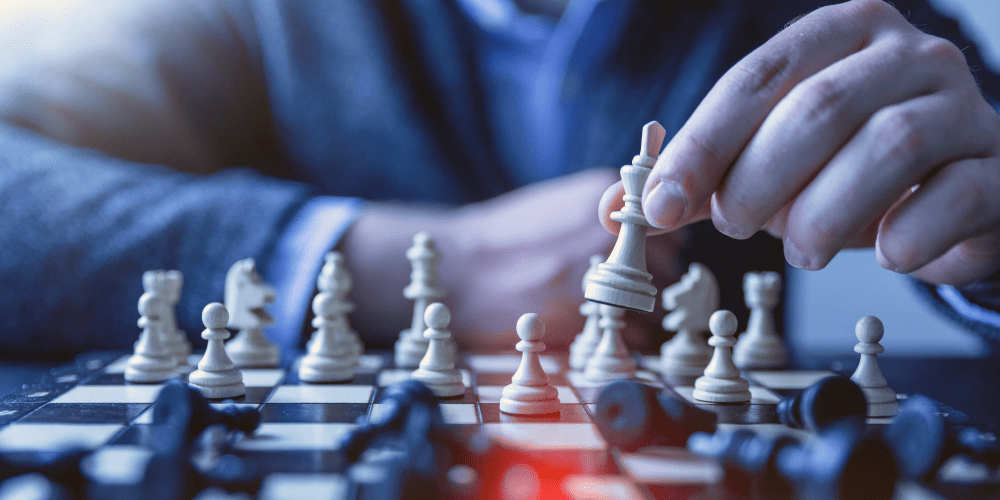
AI Decision Intelligence
Decision intelligence has been on the rise, with the job role gaining visibility beyond tech startups and into large organizations like Google and even Manchester United football club. As a function it combines data science (statistics, machine learning and analytics) with behavioral science (psychology, economics and neuroscience). Its growing demand has coincided with the adoption of data warehouses to consolidate and centralize data, creating fertile ground for machine learning. An AI model can provide an alternative to the subjective nature of other prioritization and decision tools. As humans, we tend to be affected by cognitive biases, a model on the other hand can help overcome context problems by assessing all of the inputs. It can consider product data, past experiments, qualitative feedback and broader organizational context when presenting the relevant evidence when sound boarding assumptions.
At Blok, we’re using AI to simulate product decisions. Test your assumptions and ideas, with a synthetic user base modeled on your product interaction data and psychographic frameworks. By modeling out changes, you have an evidence-based proof point to help you prioritize which experiments to implement and test live as well as evidence to help get buy-in from your team. If you're keen to see how AI can improve product decisions, book a call with us to learn more.